What are fair tests of treatments?
Not all evidence is created equal: some tests of treatments are more reliable than others.
Sometimes tests of treatments can be biased in favour of a particular result, sometimes the results occur by chance, and sometimes they are asking the wrong question in the first place.
This section of TTi is about how to tell whether a particular study is a fair test of a treatment. Only fair tests can give us reliable evidence about the effects of treatments.
In order to provide a fair test of a treatment, a study must:
Take into account the benefits of optimism and wishful thinking (otherwise known as “placebo” effects)
We know that people do better if they think they are getting an effective new treatment. Known as the placebo effect, this phenomenon has been observed consistently across many fields of human endeavour.
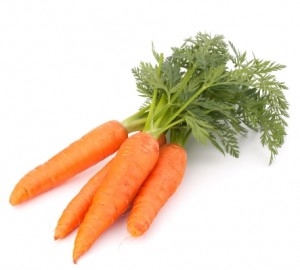
Carrots – just as good as fad diets?
For example, when a television programme invited its users to test out six different weight loss diets, they found that all of the diets were successful, including the “carrot diet”, which was not a genuine diet at all, but was made up by the programme to provide a benchmark against which to measure the other diets.
There are many different reasons behind the placebo effect, some of them well understood and others less so. We can’t predict exactly how it will affect a given test.
For this reason, we need to try to ensure that participants in any test of a treatment are unaware of which treatment they have been assigned to.
Read more.
Compare like with like
Sometimes patients experience responses to treatments which differ so dramatically from their own past experiences, and from the natural history of their illness, that confident conclusions about treatment effects can be drawn without carefully done tests.
The impaired sight of a person with cataracts is improved dramatically by replacement of the cloudy lens. Dramatic adverse effects of the drug thalidomide were revealed when women who were prescribed the drug during pregnancy gave birth to babies with extremely rare abnormalities. However, such dramatic effects of treatments, whether beneficial or harmful, are rare.
Most treatment effects are more modest, but still worth knowing about. For example, carefully done tests are needed to identify which dosage schedules for morphine are effective and safe; or whether genetically engineered insulin has any advantages over animal insulins; or whether a newly marketed artificial hip that is 20 times more expensive than the least expensive variety is worth the extra cost in terms that patients can appreciate.
In these common circumstances we all need to avoid unfair (biased) comparisons, and the mistaken conclusions that can result from them.
Usually, treatments are tested by comparing the outcomes of groups of patients who received different treatments.
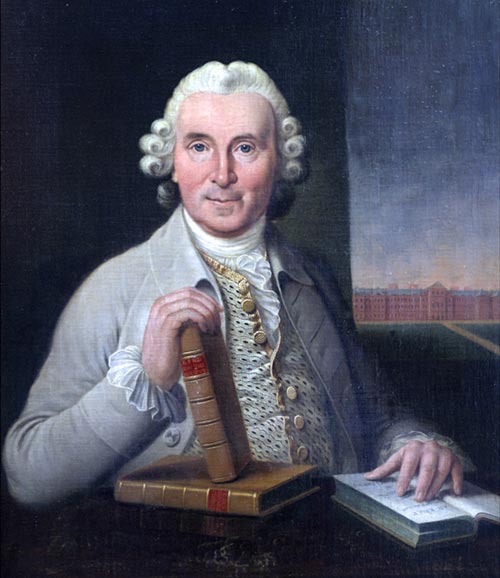
James Lind’s fair test of treatments for scurvy led to the British being nicknamed “Limeys”. Click the image to find out more.
For this to be a fair comparison, these groups need to be similar in every way apart from the treatments being compared.
For example, when James Lind compared treatments for scurvy on board HMS Salisbury in 1747, he made sure that the patients were at a similar stage of the disease, had the same basic diet and were accommodated in similar conditions.
Any difference between the participants or how they are treated can introduce bias to the test, and make it unfair.
In a fair test, all participants should be similar and treated similarly apart from the treatments being compared.
Read more.
Randomly allocate participants to the different treatments
The best way to make sure that we compare like with like is to randomly allocate participants in a study to the different treatments being compared.
In this way, as long as we have enough participants, we can be confident that the groups will be similar at the start of the trial.
In order to achieve proper random allocation we need to ensure that the people who are enrolling patients in the study don’t know what treatment is “up next”. In practice, this usually means that the random allocation is done by someone else. The participant’s treatment scheduled allocation is not known to either the patient or the researcher who decides whether they are eligible to join the trial.
A fancy way of saying this is that there has to be unbiased, prospective allocation to the different treatments being compared.
Read more
Follow up everyone who takes part in studies
After going to all the trouble of ensuring that we have comparable groups, we need to try to make sure nothing happens during the research to mess things up.
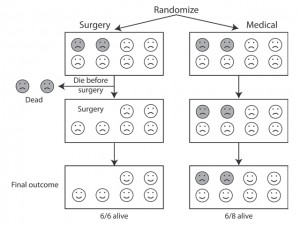
If we remove some people from one group for whatever reason, then the two groups are no longer equivalent and comparable.
If people switch treatments during the study or drop out altogether, they still need to be taken into account in the analysis. If they’re left out, bias may be introduced into the treatment comparison.
So, for example, some people may be withdrawn from the trial because of bad reactions treatment. Maybe they were more seriously ill than the others before the treatment started.
If patients experiencing bad reactions are left out of the analysis of one group but not a comparison group, then the former group is now “healthier” than the comparison group because some of the severely ill patients have been removed from it. This will bias the results of our the comparison.
All participants should be analysed in the treatment group to which they were assigned at the start of the trial.
This is known as “intention-to-treat” analysis.
Read more in “Following up everyone in treatment comparisons”.
Measure treatment outcomes fairly
We know from the placebo effect that people are more likely to report a good outcome if they think they are getting some treatments.
Therefore, fair tests of treatments shoud, wherever possible, use objective outcome measures that don’t rely on self-reports or subjective assessments.
It should also be an outcome that matters to patients, and not one that has been selected in order to make the new treatment look good!
Ideally, participants, carers and researchers should all be “blinded” to the treatment group to which patients have been assigned.
Read more in “Fair measurement of treatment outcome”.
Take account of the play of chance
Everyone realizes that if you toss a coin repeatedly it is not all that uncommon to see ‘runs’ of five or more heads or tails, one after the other. And everyone realizes that the more times you toss a coin, the more likely it is that you will end up with similar numbers of heads and tails.
It’s the same with testing treatments. Fair tests of treatments need to have enough participants to make sure that the pattern of their results aren’t just random variation.
Sometimes we don’t have enough participants in a study to rule out the play of chance. We can boost the number by combining data from similar studies. This is called “meta-analysis“. Data from such a meta-analysis in 2010 showed that tranexamic acid saves lives in trauma patients with serious blood loss.
Statistical tests can help us to determine whether the results of a test are “statistically significant“. However, statistical significance is about numbers and it isn’t the same thing as being important to patients.
Read more in “Taking account of the play of chance”.
Consider one study’s results together with those of all other relevant studies
Very seldom will one fair treatment comparison yield sufficiently reliable evidence on which to base a decision about treatment choices. Usually the findings need to be replicated in several studies before we can be confident in the evidence.
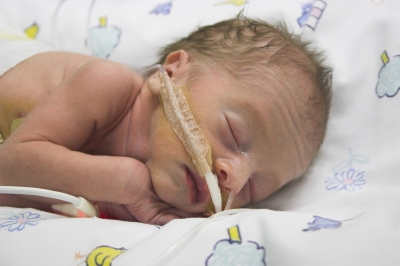
Failure to take account of previous research costs lives.
We now know that steroids save babies’ lives if they are given to mothers who are expected to give birth prematurely. But the studies showing these results were small and did not take account of previous studies. Had they done so, we would have discovered this fact earlier.
Failure to take account of previous reliable research harms patients, harms people taking part in research and wastes precious healthcare resources.
Fair test of treatments should include a systematic review of all of the relevant, reliable research that has been done before.
Read more in “Assessing all the relevant, reliable evidence“.